Evaluate at least three challenges of conducting quantitative research
Evaluate at least three challenges of conducting quantitative research
Order DescriptionEvaluate at least three challenges of conducting quantitative research. Synthesize your findings and determine an appropriate quantitative research strategy for a study on factors affecting performance of virtual teams. Analyze the issues associated with sampling validity reliability and bias within the context of this study.Conducting Quantitative ResearchNameDateAbstractThis paper looks into the process of conducting a quantitative research. To narrow down the scope of the paper the paper will look into three items. The challenges of conducting the research are addressed notably those regarding validity sampling bias and reliability. With the challenges in mind the paper will focus on formulation of a strategy to study the factors affecting performance of virtual teams. The same paper will analyse the strategy chosen and provide a verdict.Challenges of conducting quantitative researchConducting quantitative research encounters several challenges. Apart from the fact that quantitative methods tend to be complex and technical other challenges related to the research design and interpretation of the research results exists. Notably challenges exist broadly during sampling conducting research itself when analysing results and ensuring objective publishing of the interpretive results. The challenges faced present concerns about validity and reliability of the test results and the authenticity of the entire research.Challenges related to samplingFirstly sampling refers to informant election. When conducting quantitative research concerns about the size appropriateness of the informants chosen and other ethical geopolitical and socioeconomic factors of the informants present various challenges. According to Williams (2011) informant selection in quantitative research must ensure that the size of the ample is large enough to guarantee generalizability of the tested hypothesis. This means non-response of the chosen population becomes an imperative consideration. Non-response in sampling means that one will end with an inadequate sample.Komlan (2014) argues that an occurrence of a non-response can lead to overestimation of the sample or the under estimation which guarantee challenges during testing. Krishna Maithreyi & Surapaneni (2010) consequently present a sampling bias on the sample. During research occurrence of the non-response or a failure to enhance randomization of samples. In the research Krishna et al. (2010) further presented key sample biases that can occur during a quantitative research including referral or volunteer samples selection biases and measurement biases. In the process of identifying a measurable sample quantitative researchers can end up involuntarily becoming biased on the mentioned or other ways.Further any sampling bias can alter specificity of the hypothesis. According to Muijs (2011) specificity affect any numerical data to be collected and therefore becomes a major concern when choosing a quantitative design. Evidently Muijs further voices the issue of quantitative research based on forcing the sample chosen to be wholly calculable. If this is the case sometimes respondents may be forced into responding within the presented options. Chances of contrary opinions may not be included which can lead to bias and invalidity of results.Challenges related to validityAny research must show that the constructs that come out of it are valid and reflect the population or phenomena characteristics. To achieve this the researcher must endeavour to bypass several validity hurdles. Muijs (2011) observes several challenges. The challenges are those related to the construct developed criterion used the content queried or those regarding internal or external factor validities (p. 56).With regard to validity construct validity challenges emanate from the issues of theory notably those of the concepts measured and the structure of the instruments (p. 59). According to Komlan (2014) construct validity challenges come from the interconnectedness of the research work. A research sample must correspond to the instruments used and the instruments must be able to capture the items chosen for study. Concisely articulation of the items with regard to the instruments ensures that the constructs expected are the exact constructs sought for in the research which is no mean feat.Construct validity goes hand in hand with content validity which is about face sampling and item validation (Wrench Thomas-Maddox Richmond & McCroskey 2008). Achieving content validity is a challenge given the nature of objectivity and dependence on facts at the expense of narratives. Wrench and others further project that the quantitative researcher is confronted by the need to ensure that extreme areas existing in the population can be handled. In addition the possibility of controlling population and sample is at best impossible given the nature of human being in the study of an aspect of humanity. Lastly items used may not fit like-for-like with the face required or even the sample appropriateness.Criterion validity challenges are related to how the criteria chosen compares with other tests within the same class. For example if the question asked answers all issues that can lead to the same conclusion is the research in the field closing down to a similar path or inferences. According to Muijs (2011) criterion validity can be either predictive or concurrent. This means the instrument used in the quantitative research ought to guarantee prediction or relation. Topics that have wide theoretical and scholarly ubiquity present a good chance of capturing the needed criteria validity unfortunately research is not merely a move to replicate what other scholars have done but rather it is a chance to contribute to the existing body of knowledge on the topic.Ihantola & Kihn (2011) issues of generalization and variations bring internal and external validity concerns. Achieving a generalizable conclusion is a challenge in quantitative research especially given that narratives cannot be used to strengthen or items under review with relation to other existing studies.Challenges affecting reliability of researchReliability in any research is a prerequisite. Unfortunately ensuring it is not a straightforward exercise. There are concerns about how achievement of reliability is possible in a quantitative research. Usually reliability is about ensuring that replication of a similar study leads to similar results (Ihantola & Kihn 2011). Tests taken and the measured items require measurement tools which have margins of error. Muijs (2011) says that issues of reliability can come from measurement repetition or from internal inconsistencies.With repetition of measurements Komlan (2014) was of the opinion that some of the most used reliability coefficient measuring instruments like the Cronbachs alpha do not guarantee reliability. He argued that though they guarantee higher levels of probability that the measured items are reliable the whole issues of reliability stem from other sections of the research process notably in the formulation and design of questionnaires and the scales and items observed.Further reliability also stems from the way results are analyzed reported and interpreted. According to Scott (2008) on challenges of conducting research she argues that the use of accredited or suitable research best practices is not always a guarantee that research will be watertight. She reckons that even with good tests of reliability researchers can thoroughly examine or poorly interrogate results to an extent that the two gives out differing levels of conviction. Carter and Hurtado (2007) agree that eventually the issue of reliability boils down to professional and scholarly behavior whose subjectivity scars over in terms of conflicts of interests and autobiography.BiasCarter and Hurtado (2007) gave a glimpse of what bias does to research. Certain research topics or subjects have certain tendencies to use designs methods methodology analysis tools or otherwise coalescing and unique to the. For example educational research has unwritten research tendencies unique to them. People conducting education research tend to follow the codes and systems without considering whether variability is a significant concern.Bias also affects research questions design and formulation (Krishna Maithreyi & Surapaneni 2010). Such biases can alter the way the whole research process matures given that any research is interdependent of constituent parts that must marry each other at the right time. Web reliant researches face low response rates for questionnaires. This means that the non-response issue weighs heavily on the researcher during sampling. It is in this backdrop that researchers end up seeking to enhance sample size that biases occur. For example a researcher may know that conducting teenagers over the internet achieves a higher response rate that way; the researcher opts to choose a sample that is reliant on a responsive sample even when the motive is to generate a societal scope (Frippiat Marquis Wiles-portier 2010).Describing an Appropriate Quantitative Research Strategy to Study Factors Affecting Performance of Virtual TeamsBased on the numerous challenges of conducting a quantitative research it is important for the researcher to design a strategy that overcomes the challenges and enables her to test her hypotheses. Given the nature of the study seeking to establish the factors affecting performance of virtual teams it is plausible to argue that the researcher requires carrying out either a causal comparative research or an experimental research. The causal comparative research will help the researcher establish the relationship between various independent variables and a dependent variable (Williams 2011). On the other hand the experimental research will allow the researcher to introduce various controls in order to establish the effect of different independent variables on the dependent variable.For the researcher to decide which research method to use she needs to consider its vulnerability to the pitfalls discussed above and most importantly to consider its suitability in testing the hypotheses of the study. Assuming that the researcher wishes to establish which variables are statistically significant in determining the performance levels of virtual teams the method used must be able to test how social capital or group cohesiveness and knowledge sharing affects performance (Ling Kehong & Haixia 2010).In order to develop a strategy that reduces the chances of the study failing to attain credible results I will assume that the researcher will utilise either a causal comparative research or the experimental research. In either case the researchers strategy must enable her study to attain credibility. Towards this end it is important to develop a strategy that overcomes major challenges of conducting the research.Overcoming the issues of samplingSampling is a critical process in undertaking a quantitative research with the potential of causing various credibility issues. According to Ihantola and Kihn (2011) errors committed during sampling could result in loss of reliability of the study by influencing its external validity (p. 7). When a researcher selects a sample size she must be careful to select a sample size sufficient to represent not only the accessed population but also similar populations in other settings (Ibid). As observed by Carr (1994) the researcher must refrain from the temptation of using purposive or convenient samples if her study is to be valid enough to warrant generalization of the findings.The strategy here is to ensure that the researcher maintains the validity of the study by ensuring that sampling problems due to such issues as non- responsiveness are minimal and well compensated. According to Muijs (2011) the sample size is subject to time and financial constraints implying that the researcher may be unable to use a sample size that is big enough. In this regard the sample selected should be as representative of the population under study as possible. Muijs (2011) proposes ensuring that the researcher investigates those variables she expects to have more effect on the dependent variable.The researcher therefore is able to minimize the problems of sampling by ensuring that the research design is set in a manner that narrows down the variables under study. For instance the researcher might narrow down the research to focus on factors affecting performance of virtual teams through variables that affect group cohesiveness and knowledge sharing. Additionally the researcher will aim at selecting a sample size that is big enough to cover issues of non-responsiveness thus enabling her to draw a more representative sample. Ensuring the randomness of the sample is also important.Designing a questionnaireCritical to the research process is the data collection stage. Equally critical is acknowledging the high vulnerability to errors of the stage and to plan on how to minimize the possible errors. According to Frippiat Marquis Wiles-Portier (2010) sending questionnaires to online respondents have enabled improvement of question designs. Consequently a researcher is able to design questionnaire questions in a manner that proves simple and interesting to respondents while adhering to a validity criterion. To avoid situations where researchers ignore the soft data collected (Carr 1994) it is important to use analytical methods that allow the testing of every piece of data collected that is relevant to the study.In order to collect data relevant to testing the factors affecting performance it is critical to include questions that tests the expected relationships. In this regard the researcher may in addition to testing trust issues; other factors include cooperation and competition (Baruch & Lin 2012). The researcher should utilize a strategy such that the questions asked in the questionnaire are quantifiable (Frippiat et al. 2010). Questions should therefore allow coding or be framed in a way that gives numerical data.Another aspect of the questionnaires that the researcher must consider is the susceptibility of the respondent to give answers that are only convenient to him. Frippiat et al. (2010) observes that including difficult questions or complex and long procedures in the filling of the questionnaires especially in online surveys may increase satisficing (p. 305). To enable the respondents to complete the surveys the researcher should find ways to motivate them especially by making sure the questions are simple and the process captivating.By receiving credible responses from the questionnaire the researcher will be able to draw data that is usable in testing the hypotheses. Where the researcher adhered to a superior criterion validity check it is plausible to expect that the data thus acquired will be sufficient to test the hypotheses. Shuttleworth (2009) warns of the repercussions of failing to close all the gaps between the research objectives research questions the hypotheses and the data collected. Indeed Howe and Eisenhart (1990) posit that the researcher has a responsibility to ensure that the data collected provides answers to the research questions. To achieve the fit the researcher must design the questionnaires to facilitate receiving answers that contain relevant information towards her research questions.Overcoming the problems of reliabilityWith regard to reliability a working strategy in conducting this kind of research must ensure that it goes beyond the challenges. Reliability is about the size of the sample. Firstly as Black (1999) suggests the first step is to ensure that there are enough questions or enough identifying components of the constructs sought (p. 198). For example in this study one must ensure that the literature about the factors is well represented in the survey questions. Since the concern is the factors affecting performance of virtual teams existence and knowledge of such factors must be present. The factors under scrutiny ought to all be represented in the questionnaire. The core of this strategy is to ensure that errors are minimised to enhance reliability of the outcome.Homogeneity of the studied population and sample has a strong impact on the reliability of results. Because of this Shuttleworth (2009) recommends that during sampling the use of stratified random samples to ensure that the diversity of the population is captured. In this research the use of such a randomized sample enhances the accuracy of the trait measured within the population. Virtue teams can be scattered globally. Consequently an approach that captures this aspect of the population must be enhanced through randomization and sample size especially given that the study is about aspects of the population (Hopkins 2008b).Williams (2011) suggests that time consumed during the research process is vital and can affect the reliability of the results reported. During data collection time and speed required to do this must be optimum. Changing phenomena requires a short time interval between the first and the last observed. Overall research in virtual teams will also require an optimum time allowance. Although time for collection can be a week to allow respondents enough time to consider the interview time required to complete a survey must be minimum yet enough. Long questionnaires can enhance the quality of the responses as seen above but the respondents are unlikely to give it thought or carefulness in answering the prompts. Because of this the time allowed should not exceed 30 minutes to reduce respondents chances of opting out of the interview.Analytical instruments are dependent on the effectiveness of the wording of the research questions items under review and intended constructs (Black 1999). Komlan (2011) recommends that the researcher must be well informed on the exact expectation of the research normally conveyed in the research questions hypothesis and executed through specific questions in the questionnaire. Each question shall reflect an actionable and a specific outcome. For example a typical questionnaire may ask How does trust affect your performance? indicators of how can be put in terms of ratings from 1-5 scale with one meaning definitely not while five being definitely yes. Similarly qualitative analysis can help to eradicate any concern about logical flows of elemental truths.Overcoming biasData captured usually develops noise mostly in the shape of biases and inaccuracies; data cleaning is a process that is essential when one has such data. Dealing with such noise can affect the outcome of the research and therefore the recommended methods must suppress the element of noise without affecting the informants intentions (Lash Fox & Fink 2011). The writer can use Cronbachs alpha coefficients to enhance the knowledge of errors and inconsistencies. Calculation and presentation of the coefficient value and inclusion in the analysis will enhance the reliability of the measured constructs. The reason for this is because rather than have a long questionnaire that can enhance clarity of information but discourage survey responses the survey questionnaire is kept precise concise and therefore noise minimised.Equally true is that all the challenges emanate from a design that fails to arrest potential concerns at every stage. The most efficient way to reduce potential biases is through objective reasoning and consulted decisions. Through well organized literature search and synthesis the gaps identified provide questions to be asked and constructs sought. Overcoming bias is a dependent challenge whose elimination strategy is one that requires adherence to scholarly commitments.Overcoming the challenges of validityValidity is the ability of a test to produce results that explains the phenomenon under investigation (Phelan & Wren 2006). Williams (2007) emphasizes the importance of ensuring that the research is valid and credible since the sole objective of any study is to provide generalizable findings about a population. The researcher must be able to collect data that enables generalizability of her findings or that makes the relationships established between variables valid (Williams 2007 p. 66). In the case for causal comparison research the researcher must design her data collection methods to gather information that connects to the research questions. For instance in ensuring that the sampling is valid the researcher must be aware of the need to use a sample that is representative of the population. Ensuring that the sample used is random increases the chance of the selected units bearing all the tested attributes of the population.Questionnaire questions must be able to collect information usable in testing the hypotheses. Given that the respondents must respond to the questions asked it is critical of the researcher to ensure that the questions asked have quantifiable answers. In this respect the strategy would to minimize the questions whose answers will be subjective or ambiguous. Shuttleworth (2009) observes that the failure to develop questionnaires based on a validity criterion may lead to loss of credibility in the study or financial losses where the study was part of a market research. A superior strategy would ensure that the question asked in the questionnaires leads to collection of data usable in testing the hypothesis under investigation. Indeed the questionnaire ought to contain questions that directly links to the tests the researcher wishes to undertake on the hypotheses. The hypotheses being tested must also be able to meet the objective of the study by being sufficient to give inferences on the relationships being tested (Shuttleworth 2009).For example if trust is hypothesized to be a factor influencing performance respondents must respond to questions that examines issues of trust in virtual teams performance if not trust itself (Aubert & Kelsey 2003). The hypotheses must be able to test for relationship between trust and performance of virtual teams. The researcher needs to ensure she controls the responses in a manner that allows them to present a relationship between independent variables and the dependent variable (Ihantola & Kihn 2011). In this regard it is important for the researcher to ensure that the responses expected from the questionnaires can develop a connection between trust or trust issues and virtual teams performance.The issues of validity emerge in a research early on and requires proper planning to ensure that each stage remains in tandem with the bigger commitment to providing valid findings. Hopkins (2008) indicates that where the research carried out is significant; there is often the need to carry out a pilot study in order to identify all the aspects of the study and to prepare for challenges that become apparent. In carrying out a study on performance of virtual teams it is critical to carry out a pilot study in order to familiarize with the population and to determine the appropriate sample size. As Ihantola and Kihn (2011) adduces the validity of a study determines whether other scholars can use its findings to draw inferences about the whole population under investigation. A valid study will have to be able to present findings that can be useful in describing characteristics of the population.ConclusionConducting a quantitative research requires deliberate commitment to various standards in order to provide new knowledge to a specific field. While undertaking the research a researcher must overcome numerous challenges in order to provide not only reliable findings but also to ensure their validity. Pitfalls to conducting a valid research may arise from various stages in the research process such as sampling and data collection. Sampling process is critical to the research process and the researcher must be careful to minimize errors in the stage. Possible solutions to sampling problems include avoiding convenience sampling and allowing for unresponsive respondents during data collection. The sample size must be big enough and drawn from every domain of the population. In data collection stage it is important to ensure that the method used allows respondents to give clear answers and eliminates or reduces cases of satisficing. A quantitative researcher must aim towards drawing valid findings by observing a pre-planned validity criterion. To ensure the findings are valid the sampling data collection and the data analysis processes must all adhere to validity criterion and be free of bias.ReferencesAubert B. A. & Kelsey B. L. (2003). Further understanding of trust and performance in virtual teams. Small group research 34(5) 575-618.Baruch Y. & Lin C. P. (2012). All for one one for all: Coopetition and virtual team performance. Technological Forecasting and Social Change 79(6) 1155-1168.Black T. R. (1999). Doing quantitative research in the social sciences: An integrated approach to research design measurement and statistics. Thousand Oak CA: Sage Publications.Carr L. T. (1994). The strengths and weaknesses of quantitative and qualitative research: what method for nursing? Journal of advanced nursing 20(4) 716-721.Carter D. F. & Hurtado S. (2007). Bridging key research dilemmas: Quantitative research using a critical eye. New directions for institutional research 2007(133) 25-35.Creswell J. W. (2009). Research Design Qualitative Quantitative and Mixed Methods Approaches (3ndEd.). CA: Sage publications.Frippiat D Marquis N. Wiles-Portier E. (2010). Web Surveys in the Social Sciences: An Overview. Population 65(2) 285-311.Hopkins W. G. (2008a). Quantitative research design. Sport science 4(1) sportsci.org/jour/0001/wghdesign.html.Hopkins W. G. (2008b). Research Designs: Choosing and Fine-tuning a Design for Your Study. Sport science 12 12-21 (sportsci.org/2008/wghdesign.htm).Howe K. & Eisenhart M. (1990). Standards for qualitative (and quantitative) research: A prolegomenon. Educational researcher 19(4) 2-9.Ihantola E. M. & Kihn L. A. (2011). Threats to validity and reliability in mixed methods accounting research. Qualitative Research in Accounting & Management 8(1) 39-58.Jawadi N. & Bonet-Fernandez D. (2013). An examination of the factors influencing relationship building and performance in virtual R&D project teams (No. 2013-040).Komlan J. (2014). The challenge of conducting a quantitative business research: Analysis of issues with survey design sampling validity and reliability.Krishna R. Maithreyi R. & Surapaneni K. M. (2010). Research bias: a review for medical students. J Clin Diagn Res 4 2320-2324.Lash T. L. Fox M. P. & Fink A. K. (2011). Applying quantitative bias analysis to epidemiologic data. Springer Science & Business Media.Ling S. Kehong H. & Haixia P. (2010). Essential factors of affecting knowledge sharing in virtual teams. In Proceedings of the Seventh International Conference on Innovation & Management (pp. 1080-1083).Muijs D. (2011). Doing quantitative research in education with SPSS. Thousand Oaks CA: Sage publications.Phelan C. and Wren J. (2006). Exploring Reliability in Academic Assessment. Uni Office of Academic Assessment (2005-06). Retrieved on 6 February 2015 from https://www.uni.edu/chfasoa/reliabilityandvalidity.htm.Scott J. (2008). Children as respondents: the challenge for quantitative methods. In Christensen P. & James A. (Eds.) (pp. 87-109). Research with children: Perspectives and practices. Routledge.Shuttleworth M. (2009). Criterion Validity: Criterion validity assesses whether a test reflects a certain set of abilities. Experiment-resources.com.Williams C. (2011). Research methods. Journal of Business & Economics Research (JBER) 5(3).Wrench J. S. Thomas-Maddox C. Richmond V. P. & McCroskey J. C. (2008). Quantitative research methods for communication: A hands-on approach. Oxford University Press Inc.
"You need a similar assignment done from scratch? Our qualified writers will help you with a guaranteed AI-free & plagiarism-free A+ quality paper, Confidentiality, Timely delivery & Livechat/phone Support.
Discount Code: CIPD30
WHATSAPP CHAT: +1 (781) 253-4162
Click ORDER NOW..
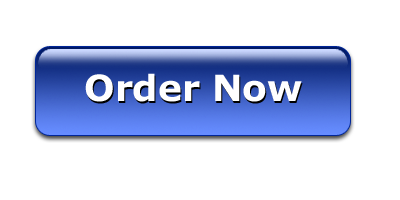